LinkedIn DARWIN¶
Introduction
Context: LinkedIn generates massive data, used by data scientists (DS) and AI engineers (AIE) for various products (job recommendations, personalized feed).
Problem: Historically, DS/AIE used diverse tools for data interaction, EDA, experimentation, and visualization.
Solution: DARWIN (Data Science and Artificial Intelligence Workbench at LinkedIn), a unified “one-stop” data science platform.
Scope of DARWIN: Goes beyond Jupyter notebooks to support the entire DS/AIE workflow.
Motivation for building a unified data science platform
Pre-DARWIN Productivity Challenges:
Developer Experience/Ease of Use:
Context switching across multiple tools.
Difficult collaboration.
Fragmentation/Variation in Tooling:
Knowledge fragmentation.
Lack of easy discoverability of prior work.
Difficulty sharing results.
Overhead in making local/varied tools compliant with privacy/security policies.
Target Personas:
Expert DS and AIEs.
Data analysts, product managers, business analysts (citizen DS).
Metrics developers (using LinkedIn’s Unified Metrics Platform - UMP).
Data developers.
Workflow Phases & Tools to Support:
Data Exploration/Transformation: Jupyter notebooks (expert DS), UI-based SQL tools like Alation/Aqua Data Studio (citizen DS, PMs, BAs), Excel.
Data Visualization/Evaluation: Jupyter notebooks, ML libraries (GDMix, XGBoost, TensorFlow), Tableau, internal visualization tools.
Productionizing: Scheduling flows (Azkaban), feature engineering/model deployment frameworks (Frame, Pro-ML), Git integration for code review/check-in.
Building DARWIN, LinkedIn’s data science platform
Key Requirements for DARWIN:
Hosted EDA Platform: Single window for all data engines (analysis, visualization, model dev).
Knowledge Repository & Collaboration: Share/review work, discover others’ work/datasets/insights, data catalog, tagging, versioning.
Code Support: IDE-like experience, multi-language support, direct Git commit.
Governance, Trust, Safety, Compliance: Secure, compliant access.
Scheduling, Publishing, Distribution: Schedule executable resources, generate/publish/distribute results.
Integration: Leverage and integrate with other ecosystem tools (ML pipelines, metric authoring, data catalog).
Scalable & Performant Hosted Solution: Horizontally scalable, resource/environment isolation, similar experience to local tools.
Extensibility: Support for different environments/libraries, multiple languages, various query engines/data sources, custom extensions/kernels, “Bring Your Own Application” (BYOA) for platform democratization.
Key Open Source Technologies Leveraged: JupyterHub, Kubernetes, Docker.
High-Level Architecture Components:
Platform Foundations: Scale, extensibility, governance, concurrent user environment management.
DARWIN Resources: Core concept for knowledge artifacts.
Metadata/Storage Isolation: Enables evolution as a knowledge repository.
Access to Data Sources/Compute Engines: Unified window.
DARWIN: Unified window to data platforms
Supported Query Engines/Languages:
Spark (Python, R, Scala, Spark SQL).
Trino.
MySQL.
Pinot (coming soon).
Direct Data Access: HDFS (useful for TensorFlow).
Objective: Provide access to data irrespective of its storage platform.
DARWIN platform foundations
Scale and Isolation using Kubernetes:
Achieves horizontal scalability.
Provides dedicated, isolated environments for users.
Supports long-running services and security features.
Leverages off-the-shelf Kubernetes features to focus on DARWIN’s differentiating aspects.
Extensibility through Docker images:
Used to launch user notebook containers on Kubernetes.
Enables platform democratization: users/teams can extend/build on DARWIN.
Isolates environments, allowing different libraries/applications.
Supports “Bring Your Own Application” (BYOA): app developers package code, DARWIN handles scaling, SRE, compliance, discovery, sharing.
Partner Team Examples:
AIRP team’s on-call dashboard (custom front-end).
Greykite forecasting library support (input viz, model config, CV, forecast viz via Jupyter).
Mechanism: Partner teams build custom Docker images on base DARWIN images, hosted in an independent Docker registry (app marketplace).
Management of concurrent user environments using JupyterHub:
Highly customizable, serves multiple environments, pluggable authentication.
Kubernetes spawner launches independent user servers on K8s (isolated environments).
Integrates with LinkedIn authentication stack.
Manages user server lifecycle (culling inactive servers, explicit logout).
Governance: Safety, trust, and compliance:
Audit trail for every operation.
Encrypted and securely stored execution results.
Fine-grained access control for DARWIN resources.
Platform
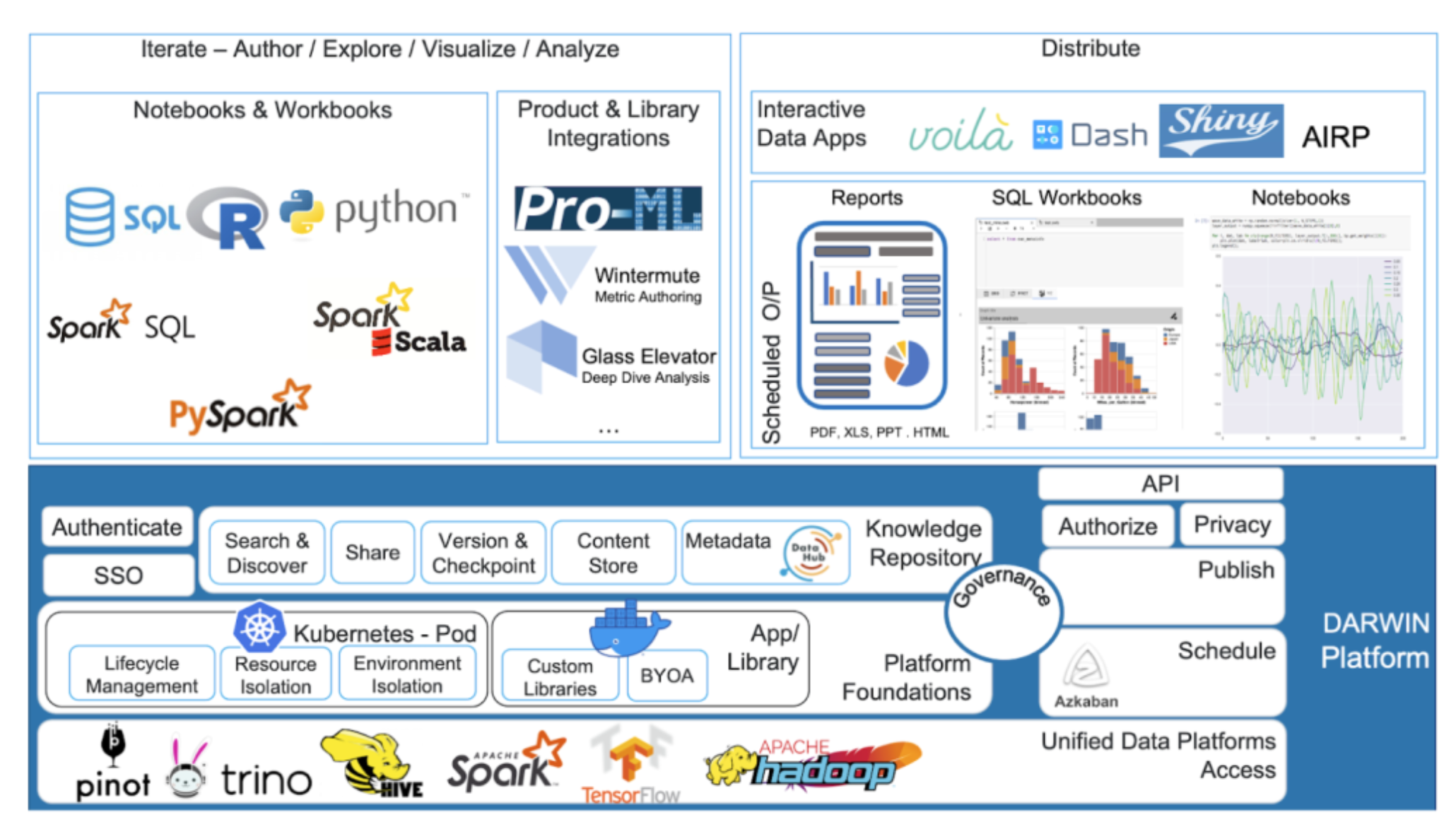
DARWIN: A knowledge repository
Vision: One-stop place for all data-related knowledge (accessing, understanding, analyzing, referencing, reporting).
Modeling as Resources:
Every top-level knowledge artifact (notebooks, SQL workbooks, outputs, markdown, reports, projects) is a “resource.”
Resources can be linked hierarchically.
Enables seamless addition of new resource types, with common operations (CRUD, storage, collaboration, search, versioning) provided generically.
DARWIN Resource Metadata and Storage:
Platform Service:
Manages DARWIN resource metadata.
Entry point for DARWIN: authN/authZ, launches user containers (via JupyterHub).
Maps resources to file blobs by interacting with Storage Service.
Stores resource metadata in DataHub for centralized management and entity relationships.
Storage Service:
Stores backing content for resources as file blobs in a persistent backend.
Abstracts storage layer choice.
User content transfer managed by a client-side DARWIN storage library (plugs into app’s content manager, e.g., Jupyter Notebook Contents API).
Enabling Collaboration:
Sharing Resources: Users can share resources (code, analysis) for learning, reuse, review. By default, shares “code only” (for privacy); owners can explicitly share “with results” to authorized users (audited).
Search and Discovery: Metadata search via DataHub.
Frontend:
Uses React.js heavily for UI (e.g., React-based JupyterLab extensions).
Provides resource browsing, CRUD operations, execution environment switching.
Key features provided by the DARWIN platform
Support for Multiple Languages: Python, SQL, R, Scala (for Spark).
Intellisense Capabilities: Code completion, doc help, function signatures for SQL, Python, R, Scala. SQL autocomplete powered by DataHub metadata.
SQL Workbooks:
For citizen DS, BAs, SQL-comfortable users.
SQL editor, tabular results, spreadsheet operations (search, filter, sort, pivot).
Future: built-in visualizations, report publishing, dataset profiles.
Scheduling of Notebooks and Workbooks:
Leverages Azkaban.
Allows parameter specification for repeatable analysis with new data.
Integration with Other Products and Tools:
Expert DS/AIEs: Frame (internal feature management), TensorFlow, Pro-ML (ongoing).
Metrics Developers: Internal tools for error/validation, metric templates, testing, review, code submission.
Forecasting: Greykite framework leverages DARWIN.
Architecture
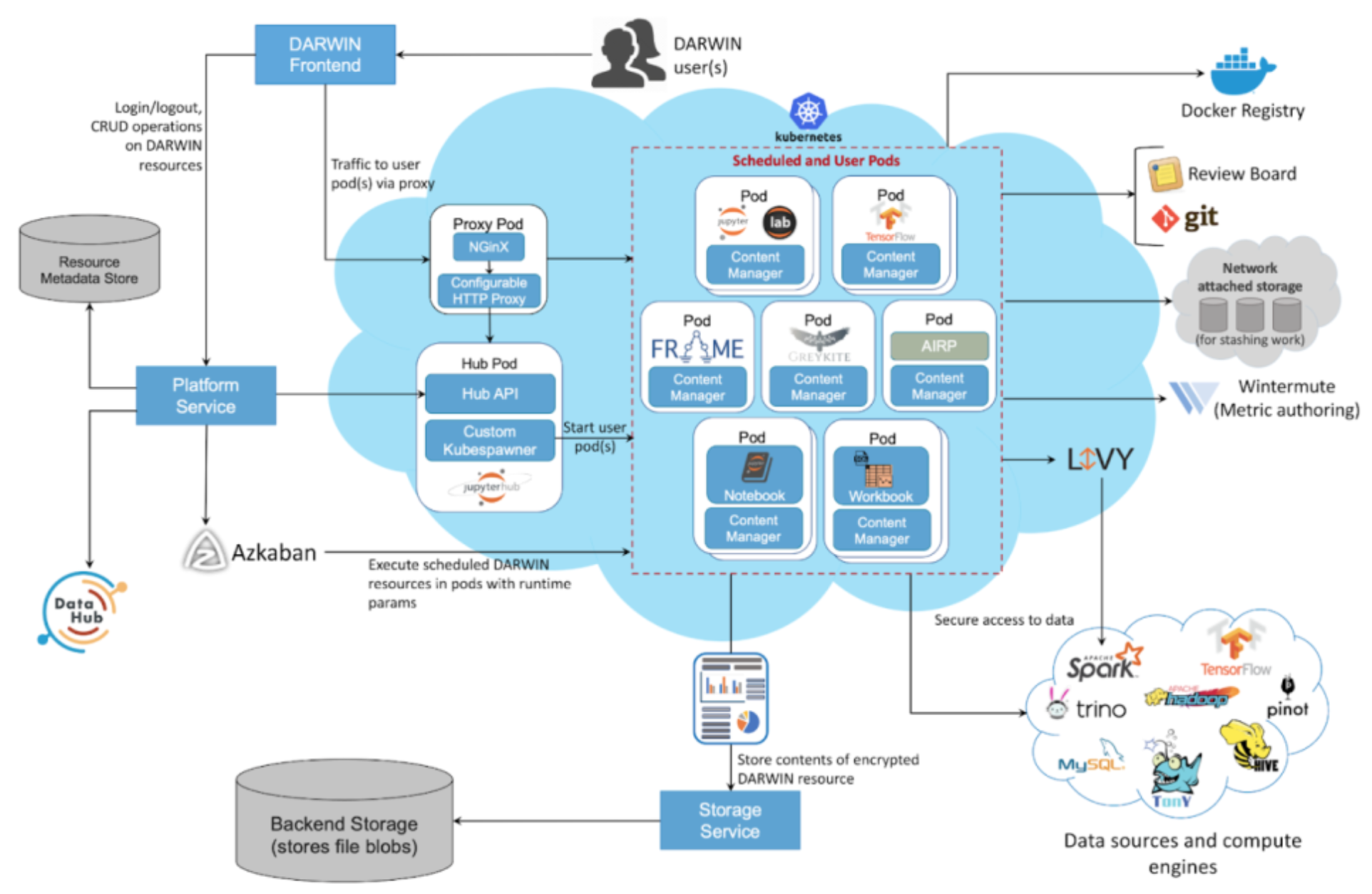
Adoption within LinkedIn
Product User Council: Formed post-launch, acts as voice of the customer for prioritization and feedback, enabling co-creation.
Scale: Over 1400 active users across Data Science, AI, SRE, Trust, BAs, product teams. >70% user base growth in the past year.
What’s next?
Publishing Dashboards and Apps: Allow authors to manage views (hide code/outputs). Host always-running apps (Voila, Dash, Shiny, custom).
Built-in Visualizations: Rich code-free viz for citizen DS (like Excel/Sheets).
Projects, User Workspaces, Version Control:
Projects as namespaces (currently public).
Plan: Manage projects on Git, enable version control.
Workspaces: Clone projects, work, commit to Git. Backed by network-attached storage.
Exploratory Data Analysis (EDA): Leverage DataHub for dataset search/discovery, schema, lineage, relationships within DARWIN.
Open Sourcing DARWIN: Eventual plan.
Ultimate Vision: Support all use cases for various personas, either natively or via integration.
Conclusion
DARWIN is evolving to meet growing/changing user needs, aiming to be the one-stop platform for DS, AIEs, and data analysts at LinkedIn.